AI & Big Data Question: What Happened to the Distinction Between Primary and Secondary Research?
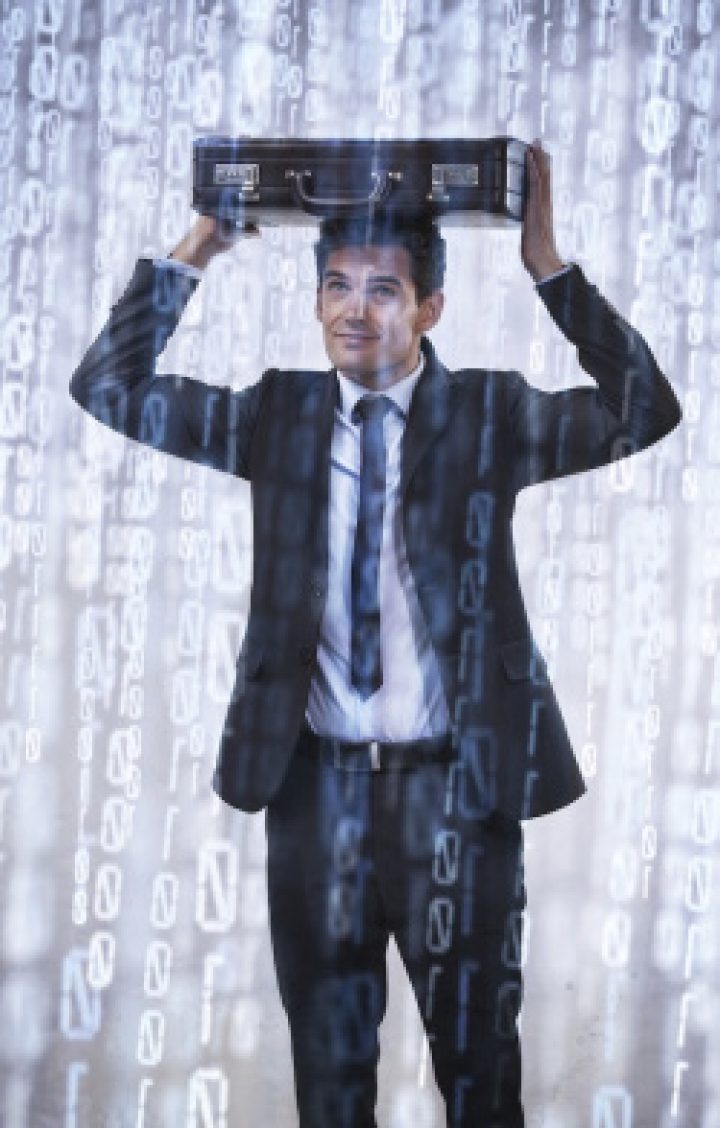
The great market hope of data analysis (now sold as “AI”) was that companies who systematically gathered “data” would create a valuable resource to guide later decisions. Lovely thought.
Look carefully at that statement, though. Because any program of “gathering data” without knowing the specific questions you want answered makes the data SECONDARY data.
It’s odd that no one is talking about this — so we’ve lost the usual cautions needed with secondary data.
The problem started as those hyping “big data” implied that bad data magically became good data once there was enough of it (in some situations there are some excellent statistics that help a lot — but you’d better be asking a lot of questions). Then AI vendors claimed they made it even better data because they have, well, the “intelligence” to do so.
What happened to the important distinction between primary and secondary in research? Let’s get the basics back on the table.
Primary data comes from primary research designed to help answer specific questions.
That means it is designed knowing opportunities for how research can assist your business in this challenge. It is also designed knowing the clear risks that research might encounter — customer biases, problems in language, problems gathering data, etc…
No secondary data can be considered primary data. And primary data is well known to be more reliable when it comes to decision making.
That said, primary research or data isn’t always an option. So there are important roles that secondary can play. But the old teaching reminds us that we ALWAYS needed to be more cautious with anything that comes from secondary data.
Secondary data is NOT primary data just as secondary research is not primary research.
There are four important secondary data sources that I’ve worked with (and there are many, many more). Read this list closely because you’ll also find it’s the list of sources for data used at the core of AI applications:
- Most data used by business is gathered in the process of doing business — it’s found data. Inside companies this includes cash register data or product sales databases.
- It may also be data which comes from research that has been done for some other purpose.
- The mass of Facebook and other data gathered online data collected about web users is also secondary data.
- There is data about people collected into massive databases which match individuals to details about their purchases, demographics, and activities. (This can go far. Many are concerned that states just might start letting student data out so that the big data sets might even include our individual Kindergarten disciplinary reports.)
Quite often, when data analysis is done, these types of secondary data are combined — attempting to match the generally known demographic data against online Facebook activity (for example).
The challenge of secondary data in AI
One reason there’s such extensive use of secondary data is that combining these data sets is the only way to make data “big enough” in order to hope to find hidden gems.
Another reason is that companies are promised that this data is cheap and might as well be used because you might learn something useful. But we must never forget that all secondary data includes hidden assumptions or is used with assumptions on the part of companies. It’s rare to see these assumptions clearly articulated as risks. Here’s a few:
- To use the data you must assume it is valid for today’s problem. This may be an error but you’ll never know that.
- The data collection process includes assumptions about the population from which it was collected OR for you to use it you must assume important things to have it be valid. Often these assumptions happen in subtle and subconscious ways.
- Secondary data must be assumed to be consistent with your interpretation of it — but it’s gathered with unknown biases or agendas. For example, we generally don’t know what data WASN’T gathered that might be more critical.
- Using secondary data means accepting whatever built in bias was assumed in interpreting the language people used. This bias may be in how questions are asked, in how open ended answers are interpreted, or in how YOU might understand the use of a term differently than the team which gathered the data.
- A great deal of secondary data is behavioral — like website actions. There are huge assumptions taken from a specific website action. Yet there are a great many reasons why someone might, for example, look at a product page on a website. Which reason can you assume? None — you need to be aware of all of them.
Why Don’t We Hear This Discussed Among Data Scientists?
The blame for this oversight needs to be placed squarely on the shoulders of the executives who bought these programs as well as the data analysis folks (now called “AI experts”) who sold them to the executives.
Still, warning alarms should have gone off throughout the corporations — especially in the research department. But they didn’t.
Warning alarms should also have gone off with consultants.
Some Direct Marketing Experience with Secondary Data
Those of us who worked direct marketing have quite a bit of experience with the data that is the foundation of data science and AI and the false promises that have been sold.
For example, I once worked with a company who did outdoor landscaping for well-to-do suburban homes. After a data project which matched customers with generally known facts about the people, I remember someone telling me breathlessly that they’d discovered our well off homeowners with families were highly likely to own mini-vans and SUVs. Um. That was a given. And we spent how much finding it out?
In another case, business reply cards matched purchasers against a magic set of segmented zip codes. We found out that buyers skewed to low population areas and that New York was the lowest per capita location of buyers. Sounds profound — and the client was ready to pull all ads from New York. Except, I ran a quick sales analysis and we sorted out that the largest single market for purchases was…New York.
Start Applying Secondary Data Caution to AI and Data Science
Please don’t misunderstand my concern. Secondary data has always played an important role in market research making corporate successes possible. Still, it remains secondary and no amount of twiddling with AI makes it anything other than secondary.
That means when we use it we must search deeply to find what it DOESN’T say and to avoid implying that it offers completeness.
It’s even more concerning to me that companies appear to have cut back on traditional primary research in order to rely on big data/AI — because of vendor and consultant promises.
Robust understanding of the world is critical to any corporate effort and key to developing the products that will deliver future demand for your company. So go forth seeking that demand. And always remember that big data and AI are secondary data. Only then can you establish the right respect for the assumptions which might be buried in what you find.
©2019 Doug Garnett — All Rights Reserved
Through my company Protonik, LLC based in Portland Oregon, I work with clients to drive innovation success with better marketing of new and innovative products and services — work which needs to start before market analysis. I also work with clients attempting to bring new life to Shelf Potatoes or take their existing products to new markets. You can read more about these services and my unusual background (math, aerospace, supercomputers, consumer goods & national TV ads) at www.Protonik.net.
Categories: Big Data and Technology, Consumer research, Innovation, Marketing Research, Uncategorized
Posted: October 10, 2019 14:18
Malpani
Posted: April 3, 2020 11:54
Privacy and Artificial Intelligence - Daizy A. Bethea Law Firm | Barristers, Solicitors and Notary Public | lawyer2call
Posted: October 22, 2020 00:06
Bringing Privacy Regulation into an AI World, Part 2: Is AI Compatible with Privacy Principles? – Design Thinking